Advanced AI Workflows: Moving Beyond Basic Chatbots
In a recent webinar, Linkenite's experts Pravin Luthada and Tisha Singh delved into the world of advanced AI-driven workflows, exploring how organizations can move beyond basic chatbots to create more sophisticated and tailored AI solutions. Let's walk through the key points of their presentation.
Introduction
In the rapidly evolving landscape of artificial intelligence, organizations are increasingly looking to harness the power of AI to streamline operations, enhance customer experiences, and drive innovation. While basic chatbots have become commonplace, there's a growing recognition that more sophisticated, tailored AI solutions are necessary to truly transform business processes. This article explores the world of advanced AI workflows, delving into why they're needed, how they work, and what organizations should consider when implementing them.
Understanding the Limitations of Basic Chatbots
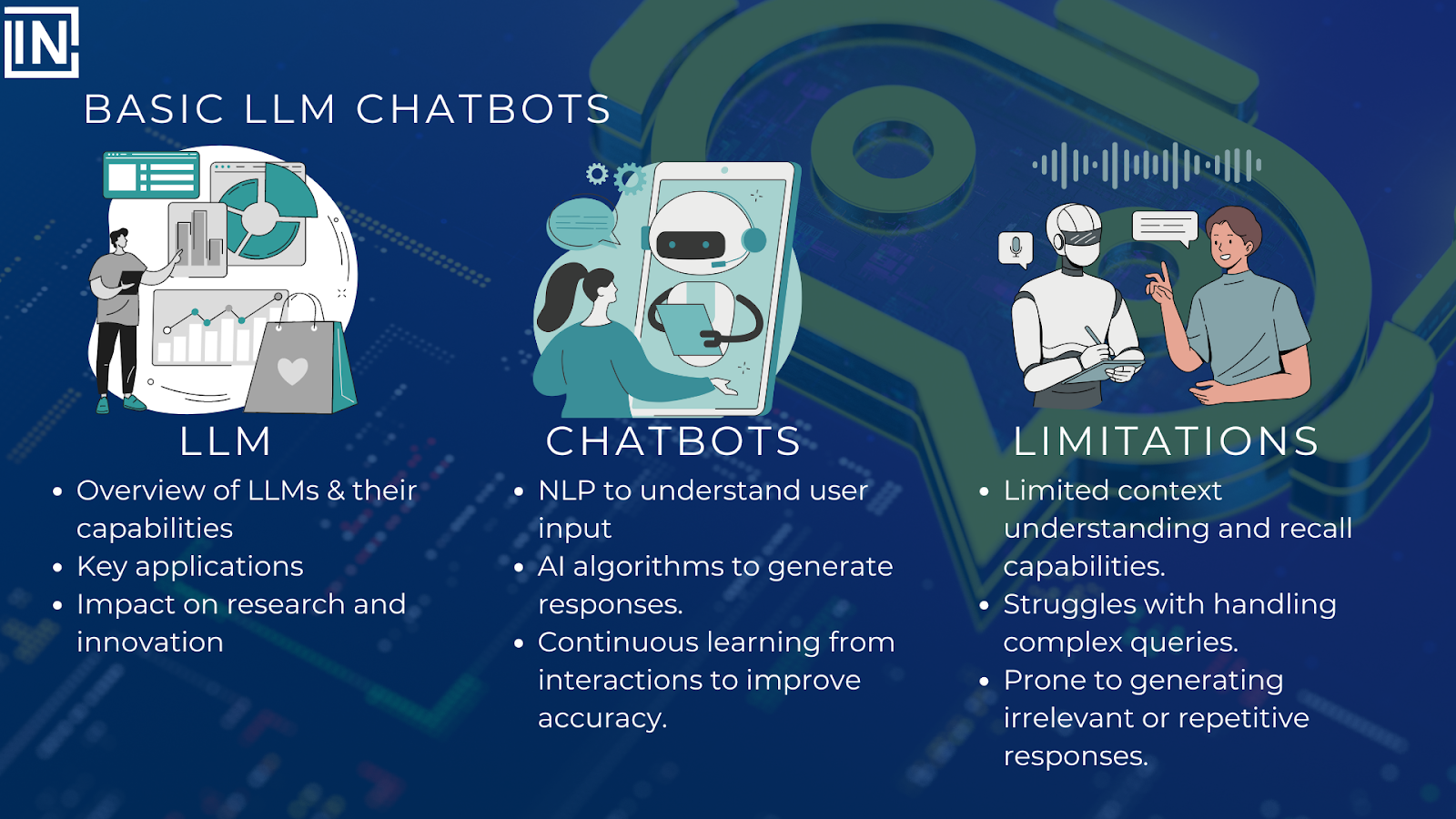
Before we dive into advanced AI workflows, it's crucial to understand the limitations of basic chatbots:
- Limited Context Understanding: Basic chatbots often struggle to maintain context over long conversations or comprehend highly specific or technical contexts.
- Difficulty with Complex Queries: Multi-step problems or queries requiring subtasks can be challenging for standard chatbots.
- Inconsistent Responses: The same query might yield different responses at different times, which can be problematic in scenarios requiring consistency.
- Lack of Specialized Knowledge: Off-the-shelf models may not have in-depth knowledge of specific industries or company-specific information.
- Potential for Irrelevant or Repetitive Responses: Chatbots may generate off-topic responses or unnecessarily repeat information.
- Limited Memory: Basic chatbots typically don't retain information from previous interactions, limiting their ability to provide personalized or context-aware responses over time.
The Need for Advanced AI Workflows
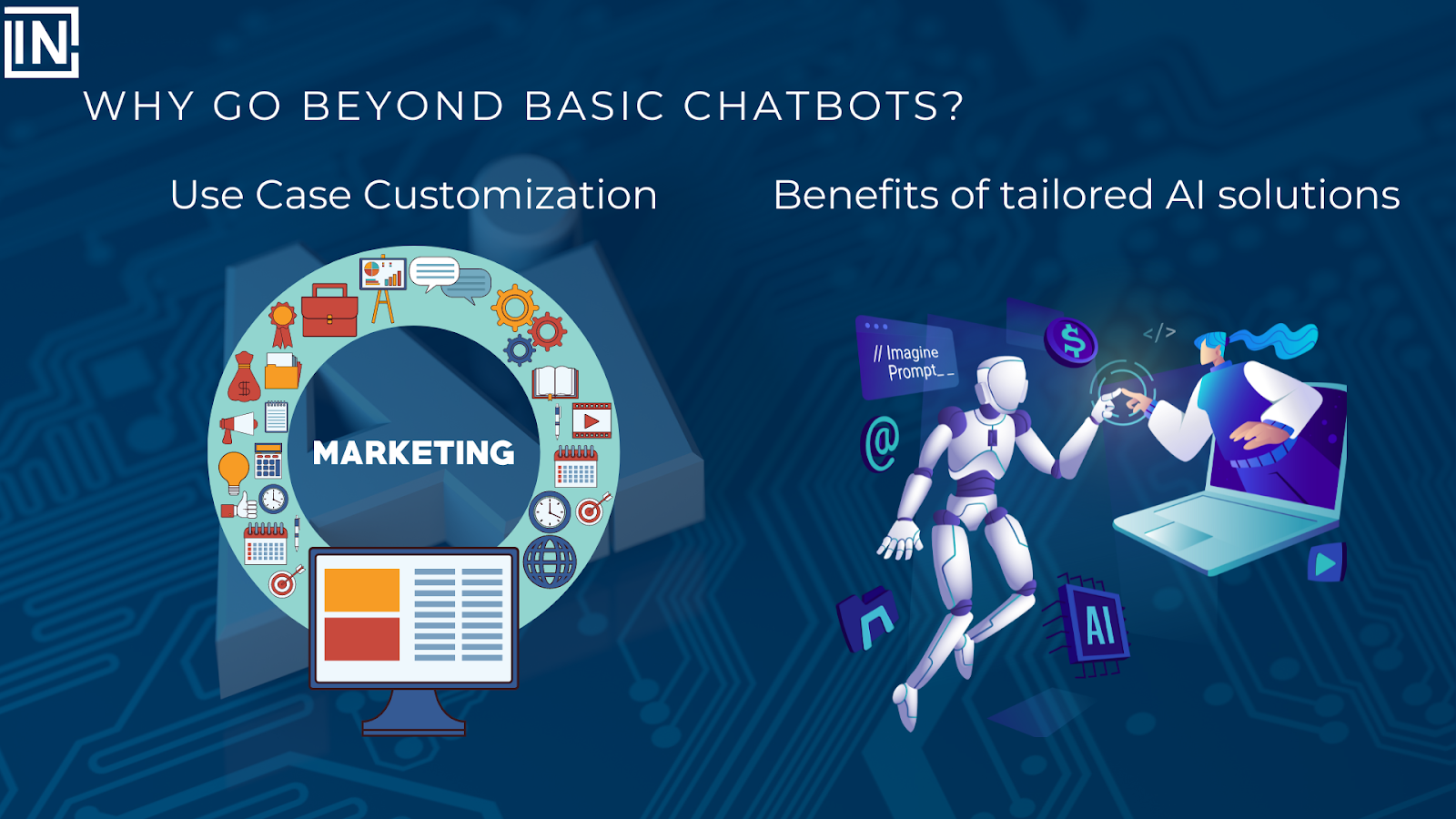
Given these limitations, organizations are increasingly turning to more sophisticated AI solutions. Here's why:
1. Customization for Specific Use Cases
Every organization has unique needs, and generic AI models often fall short in addressing them. Advanced AI workflows can be tailored to:
- Reflect Brand Identity: Ensure AI-generated content consistently aligns with a company's unique voice and tone.
- Handle Technical Content: Accurately incorporate industry-specific jargon, formulas, or data visualizations.
- Support Multilingual Operations: Maintain tone and style across multiple languages.
2. Enhanced Accuracy and Relevance
By training models on domain-specific data, organizations can significantly improve the accuracy and relevance of AI-generated responses. This is particularly crucial in fields like healthcare, finance, or legal services, where precision is paramount.
3. Improved Security and Data Privacy
Custom AI solutions allow organizations to implement specific security measures and ensure compliance with data protection regulations. This is especially important for companies handling sensitive information.
4. Seamless Integration with Existing Systems
Advanced AI workflows can be designed to integrate smoothly with an organization's existing IT infrastructure, databases, and business processes.
5. Scalability and Flexibility
As business needs evolve, custom AI solutions can be more easily adapted and scaled compared to off-the-shelf alternatives.
Implementing Advanced AI Workflows
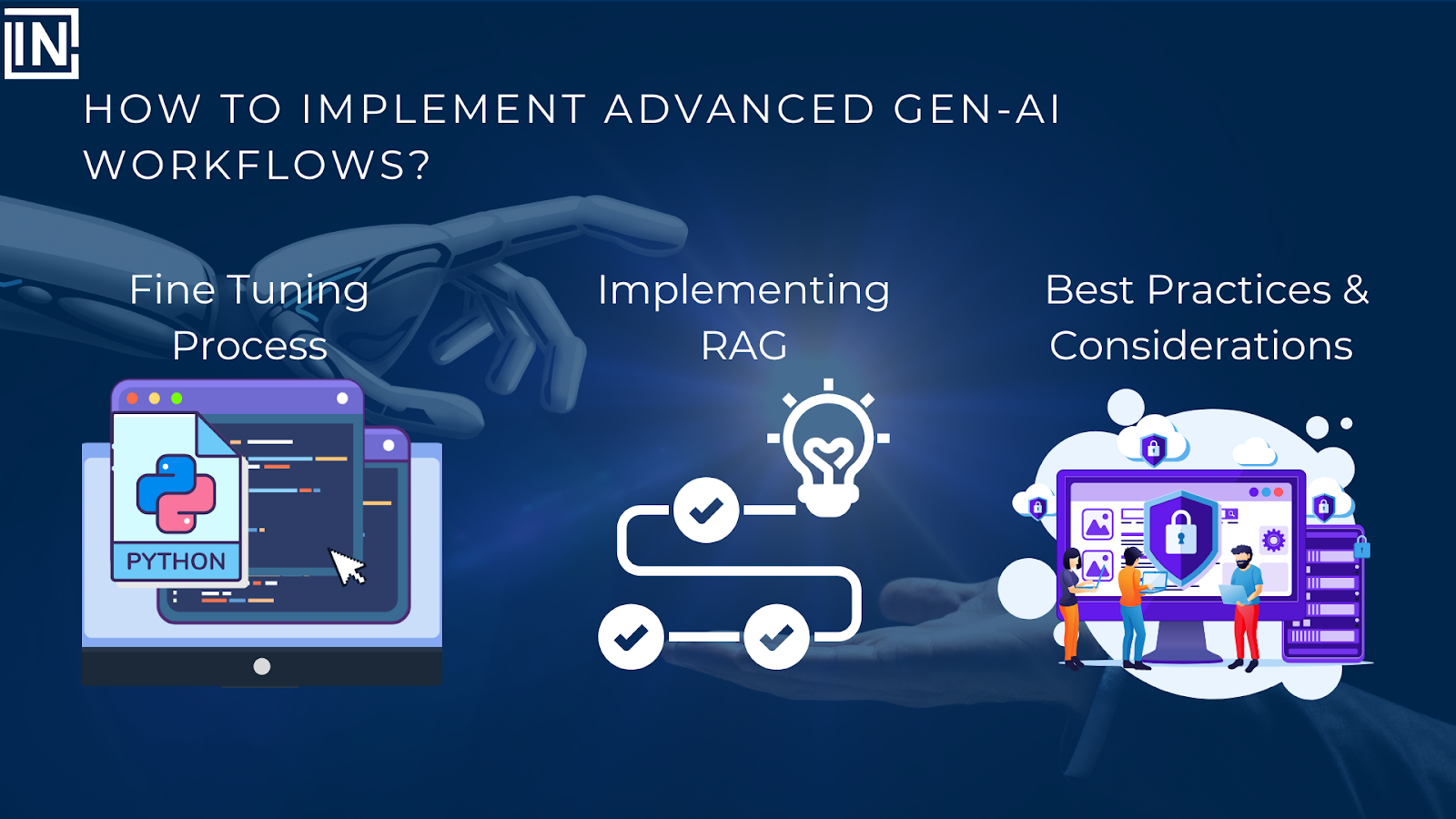
Creating sophisticated AI solutions involves two main approaches: fine-tuning existing models and implementing Retrieval-Augmented Generation (RAG).
Fine-Tuning Process
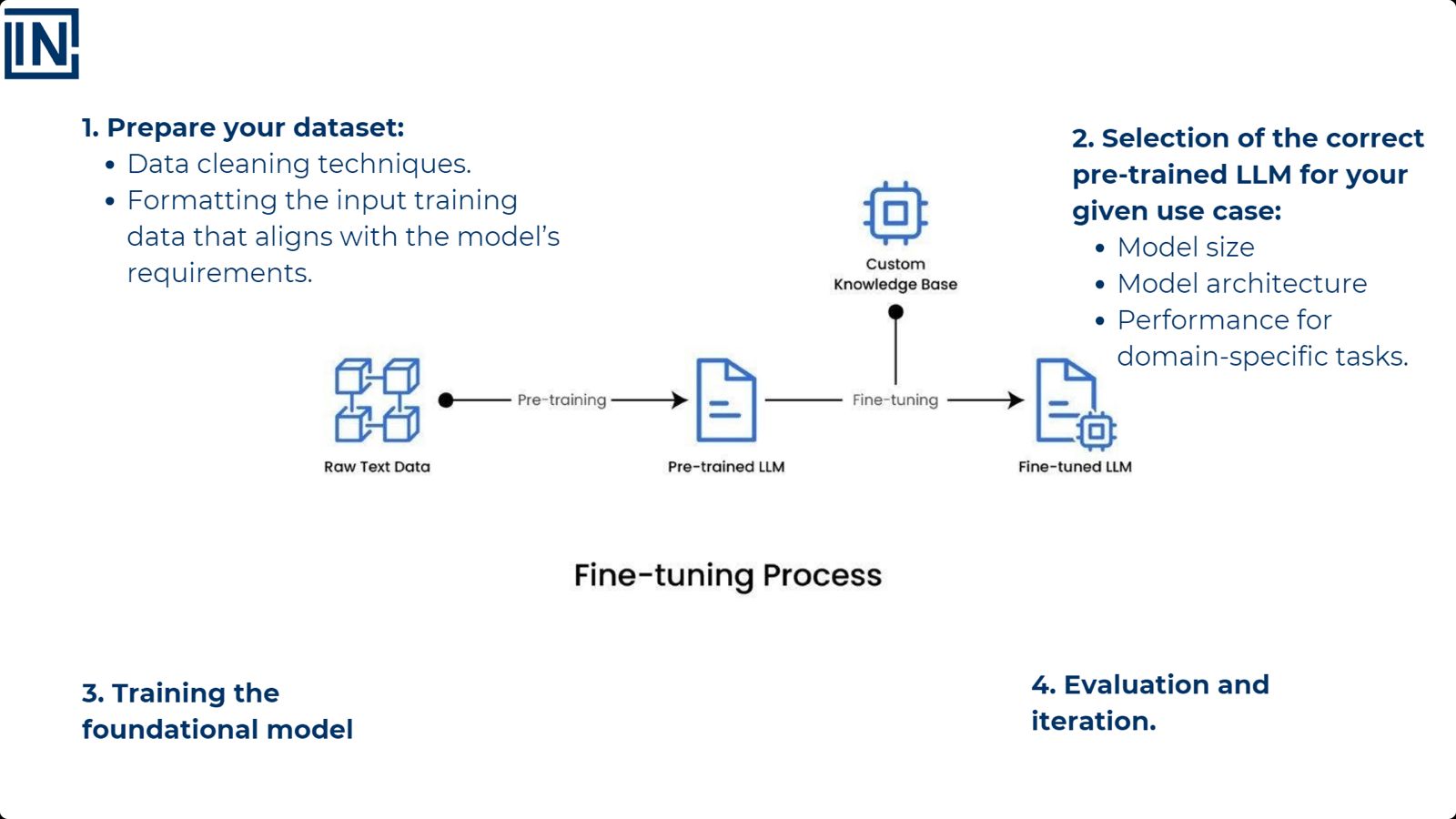
- Data Preparation: This involves cleaning and formatting input data to align with the chosen model's requirements. High-quality, relevant data is crucial for effective fine-tuning.
- Model Selection: Choosing the right pre-trained Large Language Model (LLM) based on factors like model size, architecture, and performance for domain-specific tasks.
- Training Process: This step involves further training the selected model on domain-specific data, effectively teaching it to specialize in the required area.
- Evaluation and Iteration: Rigorously testing the fine-tuned model and iterating based on performance metrics and user feedback.
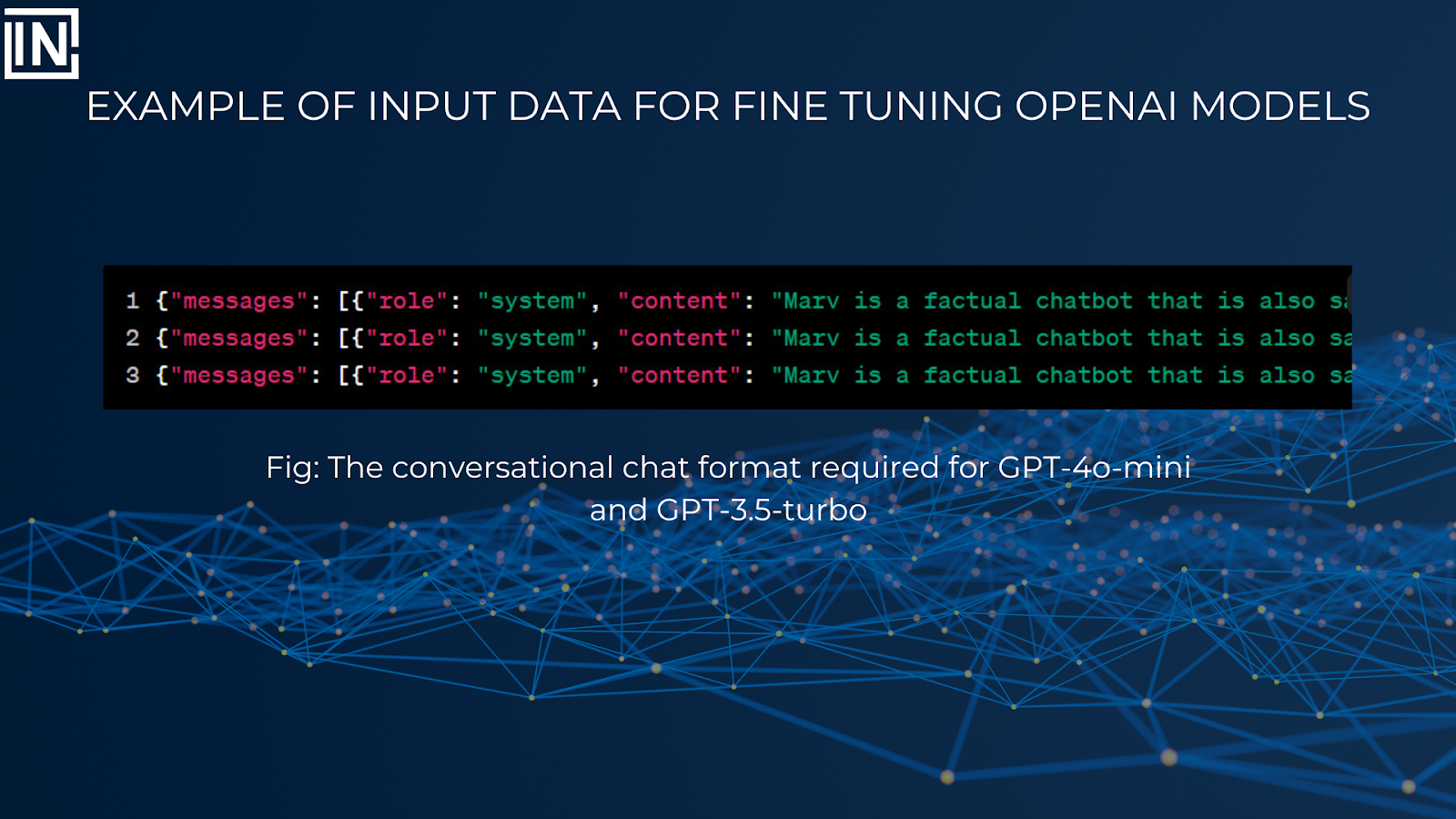
Retrieval-Augmented Generation (RAG)

RAG is an approach that combines the power of LLMs with the ability to retrieve information from external knowledge bases. This method involves:
- Setting up the Knowledge Base: Creating and maintaining a database of relevant, up-to-date information.
- Implementing the Retrieval Mechanism: Developing systems to efficiently find and extract relevant information from the knowledge base.
- Integrating with the LLM: Seamlessly combining retrieved information with the model's generation capabilities to produce accurate, context-aware responses.
Best Practices and Considerations

Implementing advanced AI workflows requires careful planning and consideration. Here are some key factors to keep in mind:
- Data Quality and Quantity: Aim for a substantial dataset (typically between 100-100,000 samples) that's highly relevant to your specific use case.
- Computational Resources: Advanced AI models require significant computational power. Be prepared to invest in high-end GPUs and ample memory and storage.
- Ethical Considerations: Address potential biases in your training data and implement safeguards against generating harmful or inappropriate content.
- Continuous Learning and Improvement: Set up systems for ongoing monitoring, evaluation, and refinement of your AI workflows.
- Human-in-the-Loop Approach: Maintain human oversight in critical processes to ensure accuracy, safety, and ethical compliance.
When to Consider Custom AI Solutions
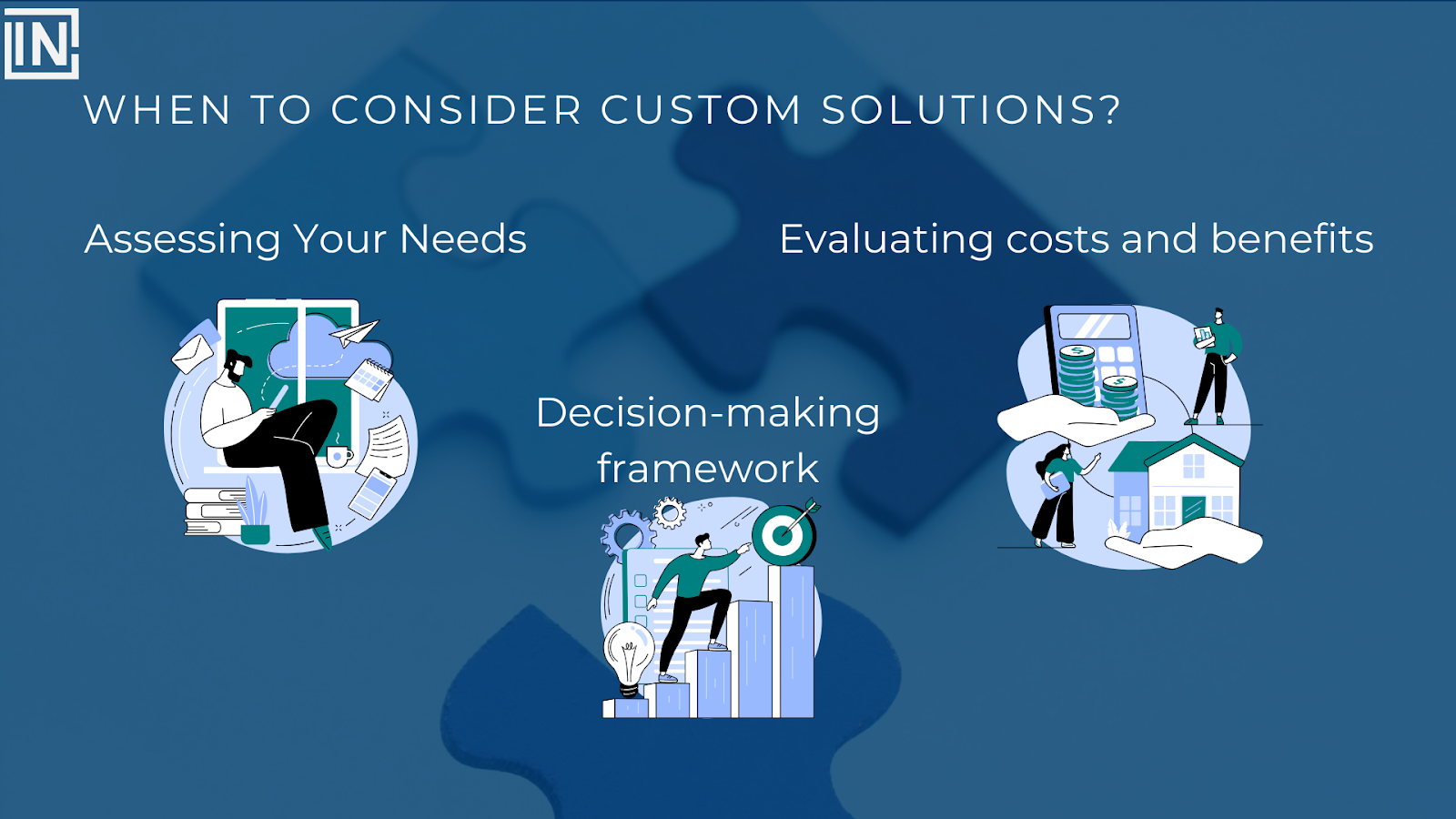
While advanced AI workflows offer numerous benefits, they're not always necessary. Consider implementing custom solutions when:
- Off-the-shelf models consistently fail to meet your specific requirements.
- You have unique data or specialized knowledge that could significantly enhance AI performance in your domain.
- The potential return on investment justifies the costs of developing and maintaining a custom solution.
- Data privacy and security requirements necessitate a tailored approach.
Conclusion
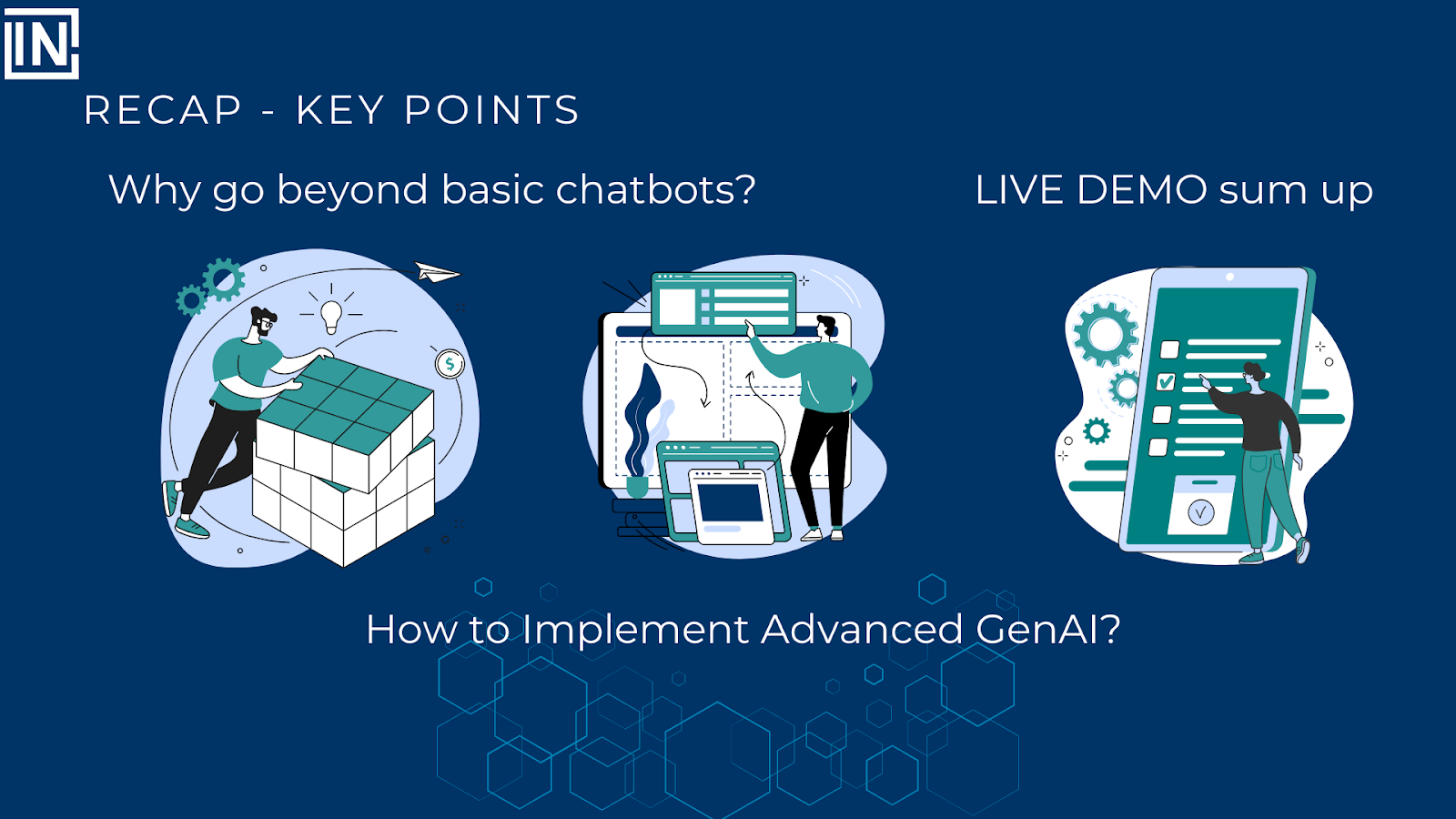
As AI technology continues to advance, the potential for custom, sophisticated AI workflows is expanding rapidly. By moving beyond basic chatbots and implementing tailored AI solutions, organizations can unlock new levels of efficiency, accuracy, and innovation. While the journey to advanced AI workflows requires careful planning and investment, the rewards in terms of improved performance, customer satisfaction, and competitive advantage can be substantial.
For organizations looking to stay at the forefront of AI innovation, now is the time to explore the possibilities of advanced AI workflows and how they can be leveraged to meet specific business needs and challenges.
